Generative AI Development
Strengthen The Development Of Your AI Solution With GenAI Data Training Capabilities
A groundbreaking GenAI development and data training software that empowers businesses to create AI solutions that focus on user privacy and security to smartly manage risk from the offset.
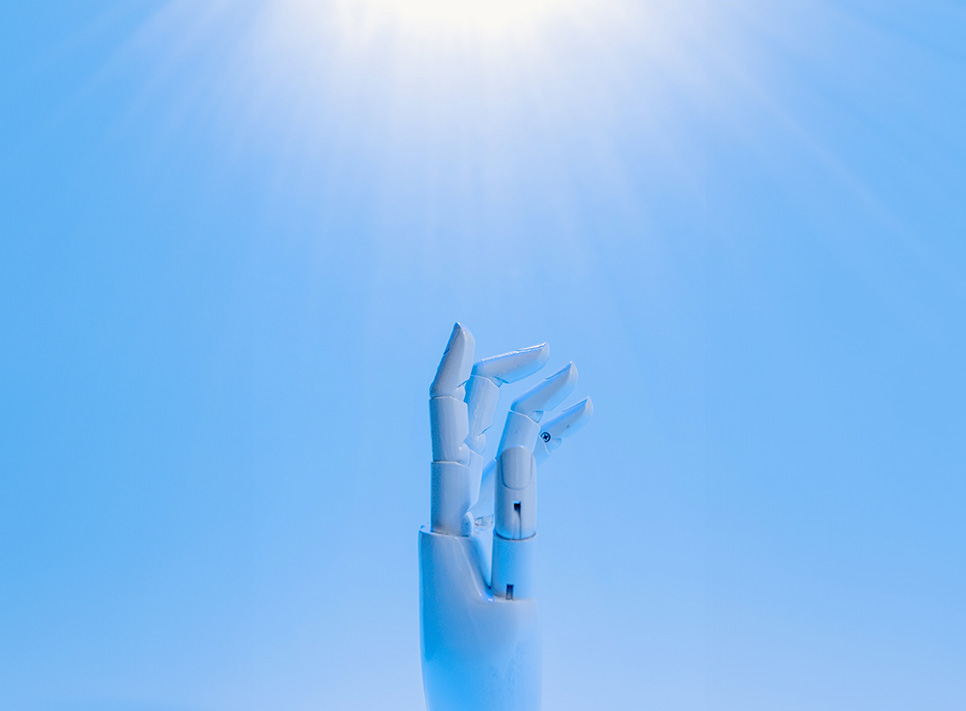
Why GenAI Data Training Essential?
As the use of AI in general and Generative AI in particular has skyrocketed, many enterprises have embarked on a journey to build and train internal AI models to aid in business decisions, productivity and workflows. These models require large volumes of actual transactional data for the AIs to produce valuable output. However, enterprises need to be very careful to not train models on sensitive or private data that either violates regulatory compliance, or presents a meaningful risk of being lost of leaked through AI output.
Empower Responsible AI Development with AI Data Training
With the increasing use of AI applications, responsible data usage is essential. Portal26’s Gen AI development and data training capabilities helps organizations protect user data, implement strict privacy controls, and manage encryption across the Gen AI development and model training lifecycle. Our solutions allow for organizations train their AI solutions from the offset to smartly manage risk and garner the most productivity growth.
The Power of Privacy Controls When Developing AI Solutions
Addressing Privacy Concerns During The Development Stage Of AI Solutions
Leverage the industry’s most robust data security and privacy platform and retain encryption while selecting and extracting AI model training data from production data. Our Gen AI data training capabilities will empower your organization to develop AI with real-world data while maintaining the highest security standards.
AI Security and Privacy Uncompromised
Portal26’s Gen AI development capabilities can output AI training data that has been cleansed of sensitive and private information according to granular data privacy and compliance policies.
Our solution supports all types of sensitive data substitution including format preserving substitutes, redaction, and traditional tokens. Additionally, Portal26 supports unlimited variations of output data via specified pipelines that maintain compliance at all times.
Portal26’s Capabilities Provides Organization's Developing AI Solutions With:

User Data Protection
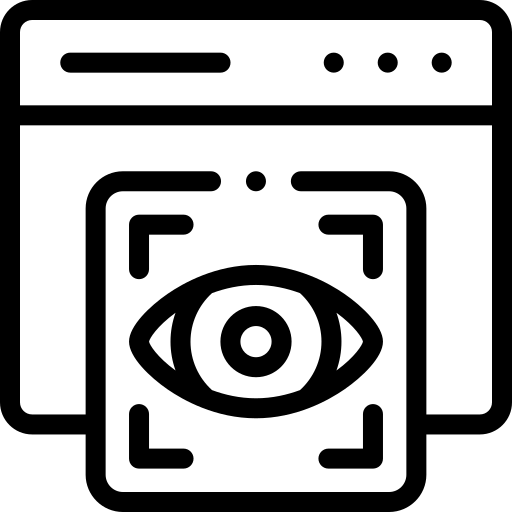
Granular Privacy Controls

End-to-End Encryption
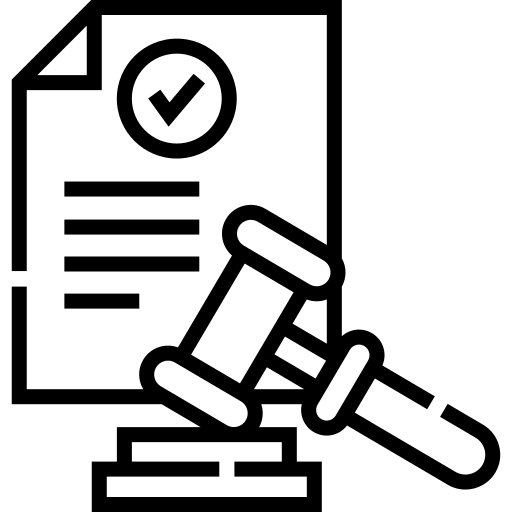
Regulatory Compliance
Experience GenAI Training Capabilities For Your AI Development Project Today
Ready to lead the charge in responsible AI development? Book a live demo with our specialists to learn everything you need to know about Portal26’s Gen AI Development and Data Training Solution. Discover how to create AI solutions that deliver innovation while protecting user privacy, security, and data.
Welcome to the future of responsible AI innovation.
Your GenAI Development FAQs
Have more questions? Contact us at info@portal26.ai or schedule a demo to get personalized answers.
When Generative AI implementation doesn’t account for ethical factors, operational risks can arise.
Unregulated inputs and lack of staff training can lead to perpetuating unintentional biases, creating discrepancies and discriminatory outcomes. This may result in reputation damage and consequently, consumer distrust. Unethical AI usage also compromises compliance with relevant regulations, which can land enterprises in unwanted legal entanglements - from legal hearings, to fines and general scrutiny. All of these factors can have a detrimental effect on the company’s bottom line, and we’ve accounted for them all with our GenAI data security platform.
Generative AI misuse can contribute to the spread of inaccurate information and distribution of deepfakes (realistic, but false, content), and these kinds of outcomes are often a result of inadequate GenAI Visibility, producing unmonitored, unregulated inputs.
Employees can also play a role in the handling and subsequent distribution of misinformation or fake content - even when it’s not intentional, and as a result of the absence of comprehensive governance measures. When GenAI usage is unregulated and unmonitored, the output results in data that is inaccurate or completely incorrect. The teams that receive these outputs may use them as a base for decisions, which has a consequent impact on the consumer at the end of the supply chain.
If a business is found to be complicit in these kinds of activities, the consequences can have a long-lasting, negative impact. From damaging stakeholder trust, to facing legal prosecution and brand image in relation to industry credibility can be tarnished.
AI usage, even during employee training, should never jeopardize user privacy. Businesses ought to take proactive measures such as removing personally identifiable information (PII) from training datasets, and using only the minimum amount of data necessary for effective learning processes to reduce the risk of privacy breaches.
The Portal26 Generative AI governance platform is devised with this value in mind, and users benefit from built-in data encryption to keep sensitive data safe during training activities and general transmission.
When the above factors are both enforced, periodic audits can prove useful, providing insights on any evident data vulnerabilities during training procedures.
When we reference undefined accountability structures around GenAI, we’re describing a scenario where responsibility for deployment of the technology hasn’t been clearly assigned between internal teams. As a result, tracking accountability for outputs becomes difficult.
Undefined accountability structures for GenAI mishaps create situations for enterprises that are difficult to learn from. Without accountability, it's hard to identify responsible parties, leading to a lack of transparency in handling issues. Ambiguous accountability can even result in legal challenges, lawsuits, and financial liabilities for the business.
To establish clear policies that encourage only responsible, purposeful AI usage, companies have to get definitive on what their ‘best practices’ are. Defining roles and responsibilities for both individuals and teams involved in GenAI development and deployment is key; and it will emphasize the importance of Generative AI visibility. This can be overseen further by having a dedicated ethics committee or a chief AI officer, and these touchpoints can guide AI usage, ensuring adherence to ethical standards.
A large language model (LLM) is an AI system built using machine learning, and it has a range of capabilities. Including recognizing and generating text. There are many types of LLM, but we’re focusing on the differences between proprietary and open-source models.
Differences in control
Proprietary models give organizations more control over the code and model architecture, though this can limit transparency. Conversely, open-source models offer greater transparency, enabling teams to inspect and modify the code - but as a result, there’s significantly less control over in comparison to a proprietary model.
Data residency
When using a proprietary LLM, organizations may have more control over where the data is stored and processed, and this can address data residency concerns. In an open-source model, data residency may be influenced by the hosting infrastructure of the OS system, leading to potential vulnerabilities as a result.
Security
In terms of security, proprietary models can be built with enhanced encryption features, whereas in open-source models, security measures depend on community contributions and might need additional customization for specific security needs.
The choice between proprietary and open-source LLMs should align with an organization's priorities, considering factors that we have outlined above. It's essential to evaluate trade-offs and select the option that best suits the organization's goals and values.